Chatbot, RAG, and GenAI: Understanding the Differences and How to Use Them
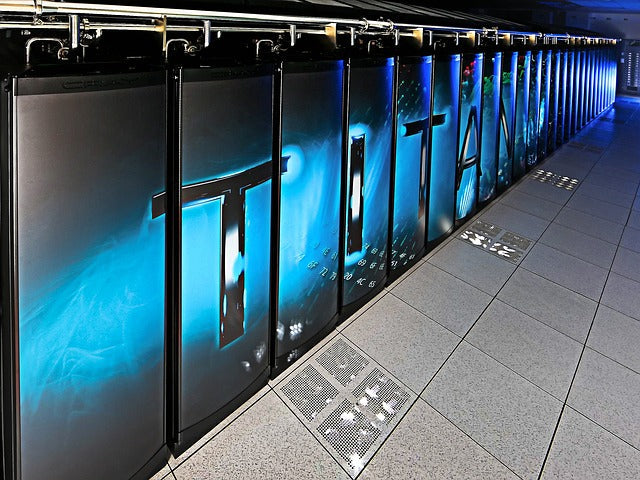
The rapid evolution of artificial intelligence (AI) has introduced a plethora of tools designed to enhance efficiency, customer service, and creativity. Among these tools, chatbots, Retrieval-Augmented Generation (RAG), and Generative AI (GenAI) stand out as significant innovations, each with distinct capabilities and applications. While they may seem similar at first glance, their differences lie in functionality, performance, and ideal use cases.
This article demystifies these technologies, explaining their unique features, strengths, limitations, and how they can be effectively utilized in various scenarios.
1. What is a Chatbot?
Definition
A chatbot is a program designed to simulate human conversation, typically to handle basic tasks or provide simple answers. It uses predefined rules or scripts and interacts with users through text or voice.
How It Works
Chatbots rely on structured databases and programmed responses to interact with users. They can operate based on:
-
Rule-Based Systems: Following predetermined pathways to answer questions.
-
Keyword Recognition: Identifying key terms in user queries to provide relevant responses.
Applications
-
Customer Support: Addressing FAQs and assisting with basic troubleshooting.
-
Banking Services: Checking account balances or initiating transfers.
-
E-Commerce: Assisting with order tracking and product inquiries.
Strengths and Limitations
-
Strengths:
-
Easy to deploy and cost-effective.
-
Suitable for handling repetitive and predictable tasks.
-
Operates 24/7, ensuring constant availability.
-
-
Limitations:
-
Struggles with complex queries.
-
Lacks contextual understanding and emotional intelligence.
-
2. What is RAG (Retrieval-Augmented Generation)?
Definition
RAG is an AI framework that combines information retrieval with text generation. It enhances AI's ability to provide accurate and up-to-date information by sourcing data from external databases before generating responses.
How It Works
-
Step 1: Information Retrieval:
-
The system searches external or internal databases for relevant data based on user queries.
-
-
Step 2: Response Generation:
-
Using the retrieved information, it generates coherent and contextually appropriate answers.
-
Applications
-
Knowledge Management: Accessing and summarizing organizational data.
-
Healthcare: Providing information from the latest medical studies.
-
Customer Support: Answering queries requiring dynamic or domain-specific data.
Strengths and Limitations
-
Strengths:
-
Delivers highly accurate and context-aware answers.
-
Integrates seamlessly with existing knowledge bases.
-
Useful for specialized and constantly evolving fields.
-
-
Limitations:
-
Relies on the quality and availability of the source data.
-
Can experience delays due to the retrieval process.
-
3. What is Generative AI (GenAI)?
Definition
Generative AI refers to AI models that create new content, whether text, images, or other data types, based on patterns learned from vast datasets. It includes advanced tools like OpenAI’s GPT, DALL·E, and similar models.
How It Works
Generative AI leverages large-scale language models (LLMs) to:
-
Analyze patterns in training data.
-
Generate creative or contextually relevant outputs that mimic human language and creativity.
Applications
-
Customer Interaction: Managing sophisticated conversations and resolving nuanced queries.
-
Content Creation: Writing articles, marketing copy, or crafting creative stories.
-
Design: Generating artwork, logos, and visual content.
Strengths and Limitations
-
Strengths:
-
Produces natural, human-like responses.
-
Adaptable to a wide range of creative and analytical tasks.
-
Highly scalable for various industries.
-
-
Limitations:
-
Requires significant computational resources.
-
Prone to inaccuracies and ethical concerns due to biases in training data.
-
4. Comparing Chatbots, RAG, and GenAI
Feature | Chatbot | RAG (Retrieval-Augmented Generation) | GenAI (Generative AI) |
---|---|---|---|
Primary Function | Automates routine tasks and FAQs | Retrieves and generates data-driven answers | Creates new content or conversational outputs |
Data Dependency | Relies on pre-programmed responses | Combines retrieval with generative models | Uses large datasets for training and output |
Complexity Handling | Handles simple, rule-based queries | Excels with dynamic and context-specific data | Handles nuanced, creative, and abstract queries |
Cost and Setup | Low cost and easy setup | Higher cost due to database integration | High cost due to computational requirements |
Use Cases | Customer service, FAQs, order tracking | Knowledge management, technical support | Content creation, advanced customer engagement |
Strengths | Fast, low-cost, and 24/7 availability | Accurate and context-aware responses | Highly flexible and capable of creativity |
Limitations | Limited to predefined scripts and responses | Dependent on source data quality | Prone to inaccuracies and ethical concerns |
5. Future Trends and Hybrid Approaches
Technological Integration
As AI technologies evolve, hybrid systems combining these tools are emerging:
-
Chatbot + RAG: For enhanced accuracy in real-time responses.
-
RAG + GenAI: For creative, data-backed outputs that combine retrieval with sophisticated generation.
Enhanced User Experience
-
AI advancements will lead to systems capable of seamless conversations that blend automation with human-like understanding.
-
Multimodal capabilities integrating text, voice, and visual content will become the norm.
Ethical and Security Considerations
-
Addressing concerns around data privacy, bias, and misinformation will be critical as these technologies scale.
6. Conclusion
Chatbots, RAG, and GenAI represent distinct but complementary advancements in AI, each with its own strengths and applications. Understanding their differences allows businesses and individuals to choose the right tool for their needs, maximizing efficiency and effectiveness.
As these technologies continue to evolve, their integration promises to transform industries, enhancing customer experiences and unlocking new possibilities for innovation.